設施農業:智慧感測【電機工程學系/溫志煜特聘教授】
論文篇名 | 英文:Real-Time Weather Monitoring and Prediction Using City Buses and Machine Learning 中文:應用城市公車與機器學習實現即時氣象監控與預測 |
期刊名稱 | SENSORS |
發表年份,卷數,起迄頁數 | 2020, 20 (18), 5173 |
作者 | Huang, Zi-Qi; Chen, Ying-Chih; Wen, Chih-Yu(溫志煜)* |
DOI | 10.3390/s20185173 |
中文摘要 | 準確的天氣數據對於規劃我們的日常活動來說非常重要。為了要檢測和預測天氣資訊,研究團隊提出了一個兩階段的天氣管理系統,該系統結合訊息處理、公車移動性、感測器和機器學習技術來提供即時天氣監測和預報。藉由本地訊息處理和公車移動性的優勢,增加動態的區域量測範圍,以提供新的感測數據。在第一階段,給定天氣感測數據,使用測試環境的溫度、濕度和氣壓值來訓練和驗證在遞歸神經網路(RNN)中的長短期記憶(LSTM)模型。在第二階段,將訓練的模型應用於時間序列天氣資料的預測。為了要評估系統效能,我們將預測的天氣數據與中央氣象局(CWB)和環境保護局(EPA)台中觀測站的實際感測數據做比較,以評估氣象預測的準確性。從結果來看,所提出的系統在天氣監測方面具有可靠的性能,且通過訓練完的模型可以很好的預測一天的天氣預報。未來可將氣象資訊模式運用於農業防災之研究,亦可提供農民進行區域性的氣象監控與預測。 |
英文摘要 | Accurate weather data are important for planning our day-to-day activities. In order to monitor and predict weather information, a two-phase weather management system is proposed, which combines information processing, bus mobility, sensors, and deep learning technologies to provide real-time weather monitoring in buses and stations and achieve weather forecasts through predictive models. Based on the sensing measurements from buses, this work incorporates the strengths of local information processing and moving buses for increasing the measurement coverage and supplying new sensing data. In Phase I, given the weather sensing data, the long short-term memory (LSTM) model and the multi-layer perception (MLP) model are trained and verified using the data of temperature, humidity, and air pressure of the test environment. In Phase II, the trained learning model is applied to predict the time series of the weather information. In order to assess the system performance, we compare the predicted weather data with the actual sensing measurements from the Environment Protection Administration (EPA) and Central Weather Bureau (CWB) of Taichung observation station to evaluate the prediction accuracy. The results show that the proposed system has reliable performance at weather monitoring and a good forecast for one-day weather prediction via the trained models. |
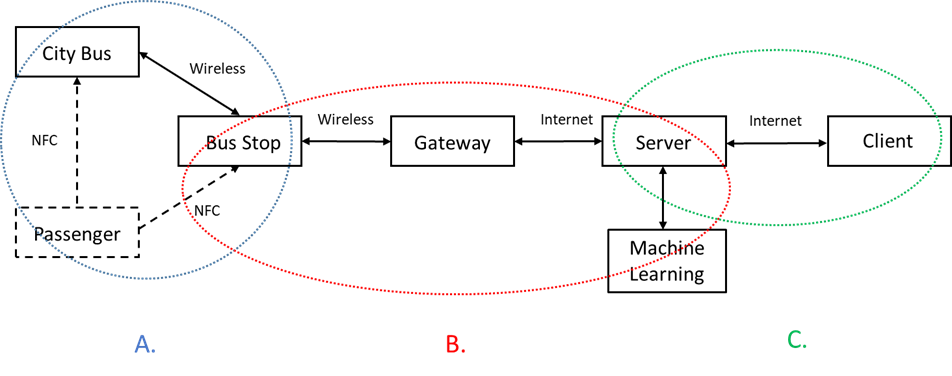
Figure 1. Intelligent bus system model for weather monitoring (left); the system architecture for real-time weather monitoring and prediction (right): the dashed lines indicate the communication links related to passengers, and the solid lines indicate the communication links between each part of the system Note that parts (A), (B), and (C) represent bus–station operations, station–gateway–server operations and server–client operations, respectively.