論文篇名 | 英文:A Novel Centrality Based Method for Visual Analytics of Small-World Networks 中文:一種新穎中心基礎之網絡可視化分析方法 |
期刊名稱 | Journal of Visualization |
發表年份,卷數,起迄頁數 | 2019,22,973-990 |
作者 | Lin, C. C. W. Huang, W. Y. Liu*, and S. F. Wu |
DOI | 10.1007/s12650-019-00582-5 |
中文摘要 | 現今需要處理和理解的網路資料變得越來越大複雜。小世界圖形具有較小的直徑、較短的節點間平均路徑長度、以及高度的叢集結構,此類圖形廣泛的應用在各種科學領域,包括社群網路、社會學、計算機科學、商業智能、和生物學。然而,傳統用於繪製小世界圖形網路的視覺化演算法常導致節點均勻的聚集成一塊,或其繪圖受限於樹形結構,從而使網路結構難以識別和分析。本研究提供一種新的視覺分析方法來改善這種情況。與以前基於生成樹的方法不同,該方法首先基於網路中心性度的度量值生成一個加權平面子網路。然後使用基於節點邊排斥力的力導向演算法來繪製此子網路,以便更好地理解此網路數據。最後,其餘的連邊放回原處以維護原始網路的完整性。實驗結果顯示,與過去方法比較,此方法在區分叢集和揭示網路中各個節點與叢集之間的關係樣式方面更為有效。此外,作為個案研究,將本研究所提方法應用於半導體晶片製造行業的數據。本研究顯示,本研究之方法讓使用者可獲得對數據有用的見解。 |
英文摘要 | Nowadays, the network data that we need to deal with and make sense of are becoming increasingly large and complex. Small-world networks are a type of complex networks whose underling graphs have small diameter, shorter average path length between nodes, and a high degree of clustering structures and can be found in a wide range of scientific fields, including social networks, sociology, computer science, business intelligence, and biology. However, conventional visualization algorithms for small-work networks lead to a uniform clump of nodes or are restricted to a tree structure, making the network structure difficult to identify and analyze. This work provides a new visual analytical method to improve the situation. Different from previous methods based on spanning trees, this method first generates a weighted planar sub-network based on the measurement of network centrality metrics. A force-directed algorithm based on node-edge repulsion is then applied to visualize this sub-network into a proper layout for better understanding of the data. Finally, the remaining links are placed back to maintain the original network’s integrity. The experimental results show that compared to previous methods, the proposed method can be more effective in differentiating clusters and revealing relationship patterns among individual nodes and clusters in the network. Furthermore, the proposed method is applied to a data of the semiconductor wafer manufacturing industry as a case study. The work shows that this new approach allows users to gain useful insights into the data. |
【學術亮點】一種新穎中心基礎之網絡可視化分析方法 2019-08-26
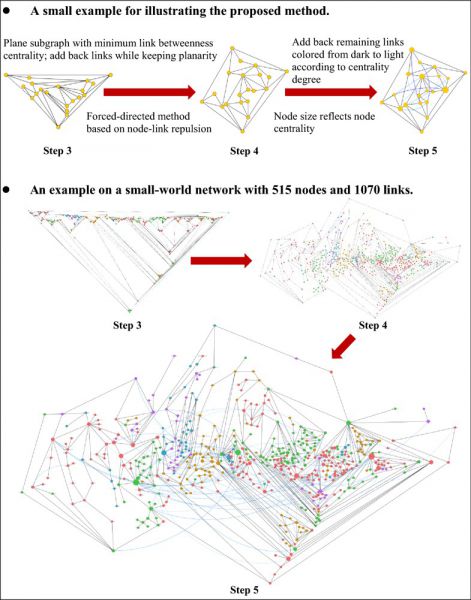
生態農業:農業精準栽培管理技術開發【森林系柳婉郁特聘教授】